Post provided by Liam MacNeil
Collecting data from thousands of biological specimens can reveal wide scale patterns, however, doing this manually is time intensive. In this blog post, Liam MacNeil describes their automated approach to data collection and the insights this provided on mudsnail morphology.
Evolution on the beach
Charles Darwin begins his masterwork On the Origin of Species (1859) with charming examples of selective breeding in dogs and pigeons, demonstrating a process (artificial selection) that generates patterns of phenotypic variation. But it was his hard labor observing barnacles which ultimately helped shape the theory of evolution by natural selection. These intertidal invertebrate offered a wealth of samples to rigorously analyze patterns of selection in nature.
Coastal zones have long been a focus for understanding ecological patterns, but this scientific history hides the relative lack of data produced and analyzed in marine benthic communities. This is surprising given that a substantial, if not a majority, of global biodiversity and biomass is harbored in marine sediments.
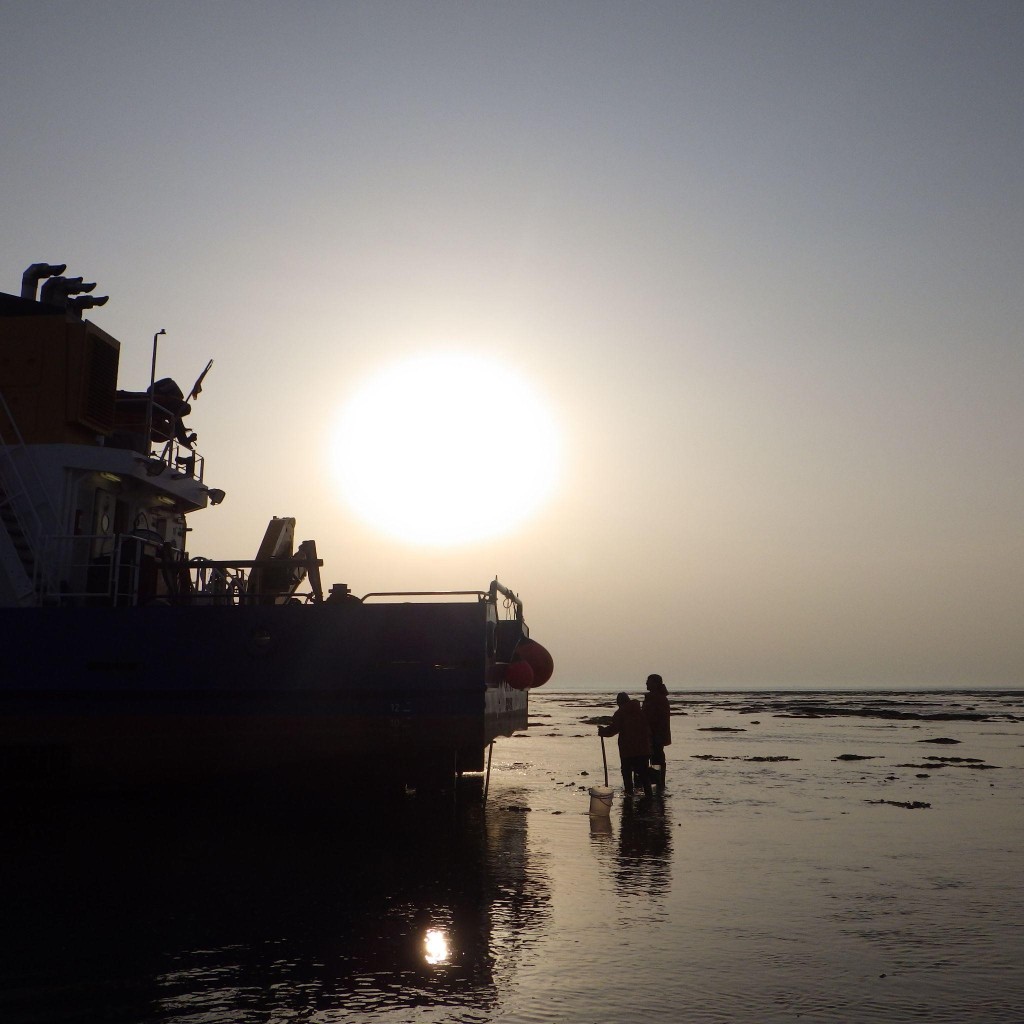
Our recent article uses a mesocosm setup, which is a model of the nearby Wadden Sea. This mesocosm permits high-frequency sampling of benthic infauna under different temperature treatments.
Sorting snail superpixels
To expedite counting and sizing of the dominant mudsnail, we apply computer vision tools to process, segment, measure, and preserve detailed metadata. These tools are all reproducible under FAIR (Findable, Accessible, Interoperable, Reusable) data protocols.
Our article demonstrates a workflow to segment mudsnails (which display complex sizes, shapes, and colours) from 2-D images and accurately measure multiple morphological properties from each individual. The basis for this is in superpixels: Representative groups of pixels based on shared low-level features that enabled fast extraction of objects by clustering superpixels together and then algorithmically segmenting clusters.

Automated size measurements proved accurate for > 4.5k mudsnails that we measured manually. When scaled across the larger mudsnail dataset, our method automatically measured > 42k mudsnails in roughly a fifth of the time taken to measure manually (~ 2 minutes per image).
Snails in the spring
We found marginal mudsnail growth which is only explained as seasonal growth in spring, and not between different temperature treatments. Suggesting that this common mudsnail, distributed throughout the northwest Atlantic coastline, shows resilience in growth up to 3 °C of ocean warming. These developments in measurement methods invite research to study mudsnail growth sensitivity under a changing climate.
Future work
The fundamental approach shown in our article can be extended beyond counting and measuring individuals. Accurately partitioning images (or videos) based on innate natural features opens possibilities to analyze responses of individual traits (e.g., elongation, roundness, color) against environmental gradients in landscapes or assessing seasonality through time. However images are collected, superpixels can help derive information about biological objects embedded within photographs. Superpixels can also be combined with deep learning models to improve representation and learning of object features.
Beyond improving image-based analyses focused on benthic ecology, our work is fully developed in the R programming language with aims to expand towards similar functionality in many open-access tools (e.g. Python). Computer vision can make quantitative monitoring possible for benthic communities, assessing impacts by species invasions, environmental stressors and broader climate changes. As we have demonstrated, advances in computer vision will continue to improve and modernize ecological studies.
You can read the full article in Methods in Ecology and Evolution here